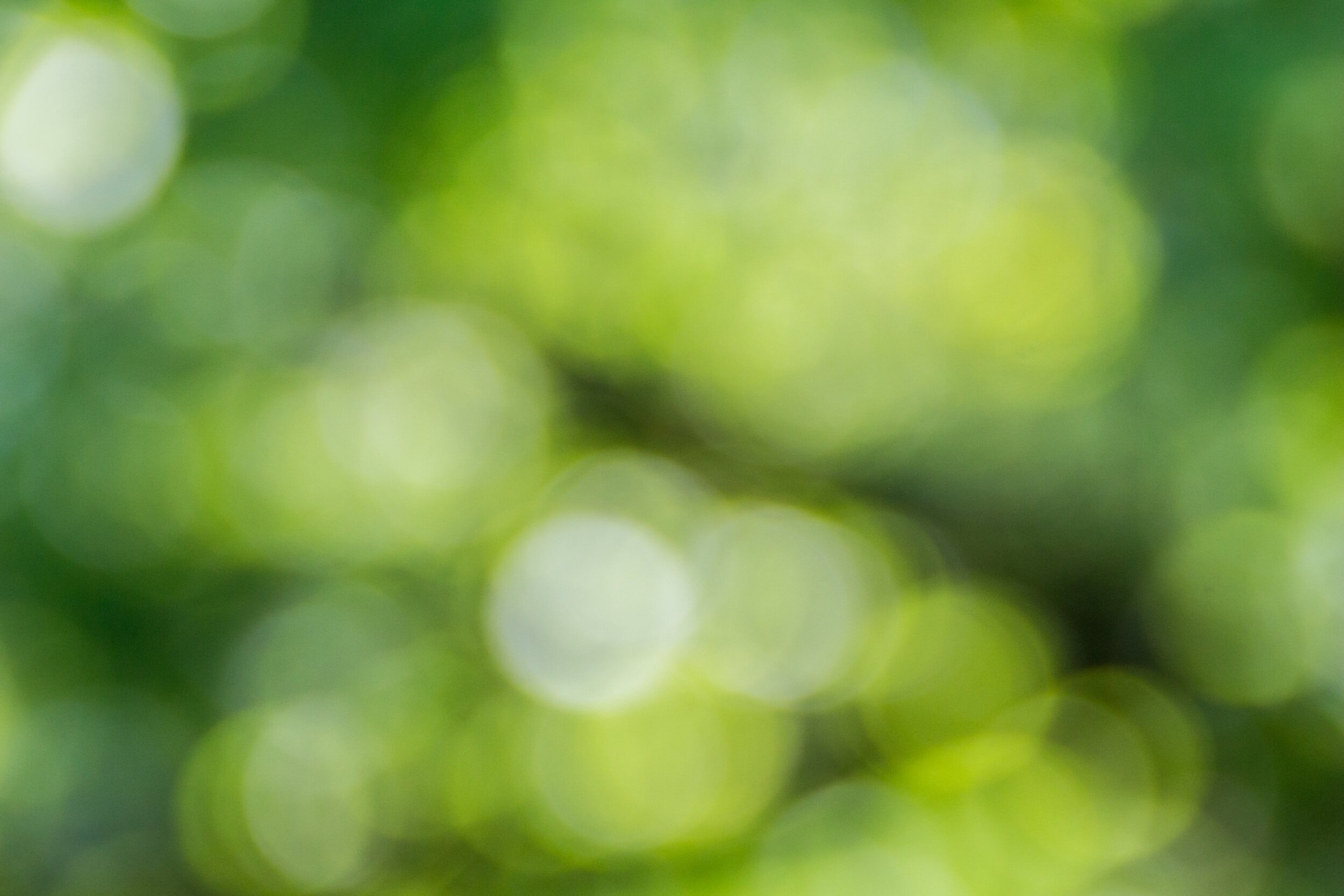
2023 Q1 | Edition 1 | Article 2
Being confidently wrong
Sometimes, the price we pay for precise data is just too high. Luckily, the human race has been collecting flawed data for decades, and know a thing or two about how to handle it.
The factory supervisor’s dilemma
Say you run a manufacturing plant and you want to know how many of the products your plant produces every day are defective. You could quality control check every product that comes off the production line, and if you’ve been put in charge of Apple phones, for example, you might well want to do that. Your entire business model is based on the premise of the product being justifiably expensive.
What if you run a mass manufacturing plant, say a car plant that produces 3,800 vehicles a day. That’s 815,00 per year and a lot to check individually. It may not be financially viable to test all of those vehicles. Think of the physical space you would need just to hold that many cars in a waiting bay while you check them, and the manpower needed.
It makes more sense to check a random sample of the cars, to get an idea of the scale of any problems with quality, say 50 cars per day. A small team could check those cars and work out the proportion of perfect to faulty cars. Let’s say 2 were found to have a fault in that sample of 50. That’s 4% of the cars. Presumably, that means that 4% of all the cars produced that day were faulty, meaning around 152 cars ( 4% of the total of 3,800 vehicles per day). Yikes!
We all have bad days
The next logical question after getting that high figure would be, ‘Was that just a bad day? Or do many of our cars come with off the production line with a hefty snagging list?’ Such a high fail rate isn’t going to be good for business.
At that point, the manager makes a simple assumption. He or she assumes that they made a mistake. And they assume if they repeat the same test the next day, they’ll probably make a mistake again, and the day after and so on. This assumption of error is what frees the manager up to get a little closer to a true number.
If you checked a sample of 50 cars every day, you may well find a lot of difference in the fail rate each day. Some days it might be just 0.1%, other days it might be 4% depending on many factors – the batch of parts used, how each machine was running or the staff who were in that day (assuming that there might still be a few humans in the mix). If you plotted all of those mistaken, inaccurate measurements on a chart, you would start to see a bell-shaped curve appear.
Getting error to work in your favour
From that chart, we can see that the average of every day was around 0.5%. That’s better! That’s still around 19 cars per day, but reasonable and only minor faults in paintwork or trim were found. Our measurement of 4% was a particularly unlucky pick. But is that 0.5% the true figure? Probably not – there is still a fair bit of chance involved.
The bell shape of the chart really matters in mathematics, because once we get data into that shape, we can do so a lot of analysis on it. We start by looking at the number of samples we took and the amount of variance between the daily failure rate and get a ‘confidence interval’ for our true average. We think that the true fail rate is somewhere between 0.2% and 1.5%. It’s not perfect, but it’s a number we can now work with more confidently and we haven’t had to employ a team of 500 Quality Assurance experts to check every car each day.
This process is called Central Limit Theorem. It’s the idea that once we can get a mean of a range of samples, we can get a little closer to estimating the true mean in the ‘population’. In this case, the population would be the daily output of 3,800 cars. This is not the world of maths you learnt as a kid at school, where there was one correct answer, and nobody could challenge it. It’s a model of mathematics that assumes that we are always a little bit wrong. But once we’re aware of our own mistakes we can start to make them work for us.
Our next article …
How do you know you know?
One of the troubles with human thinking is that a person who knows useful skills and knowledge tends to doubt their own abilities. A person who knows nothing can be hyper confident. So how do you know you know?