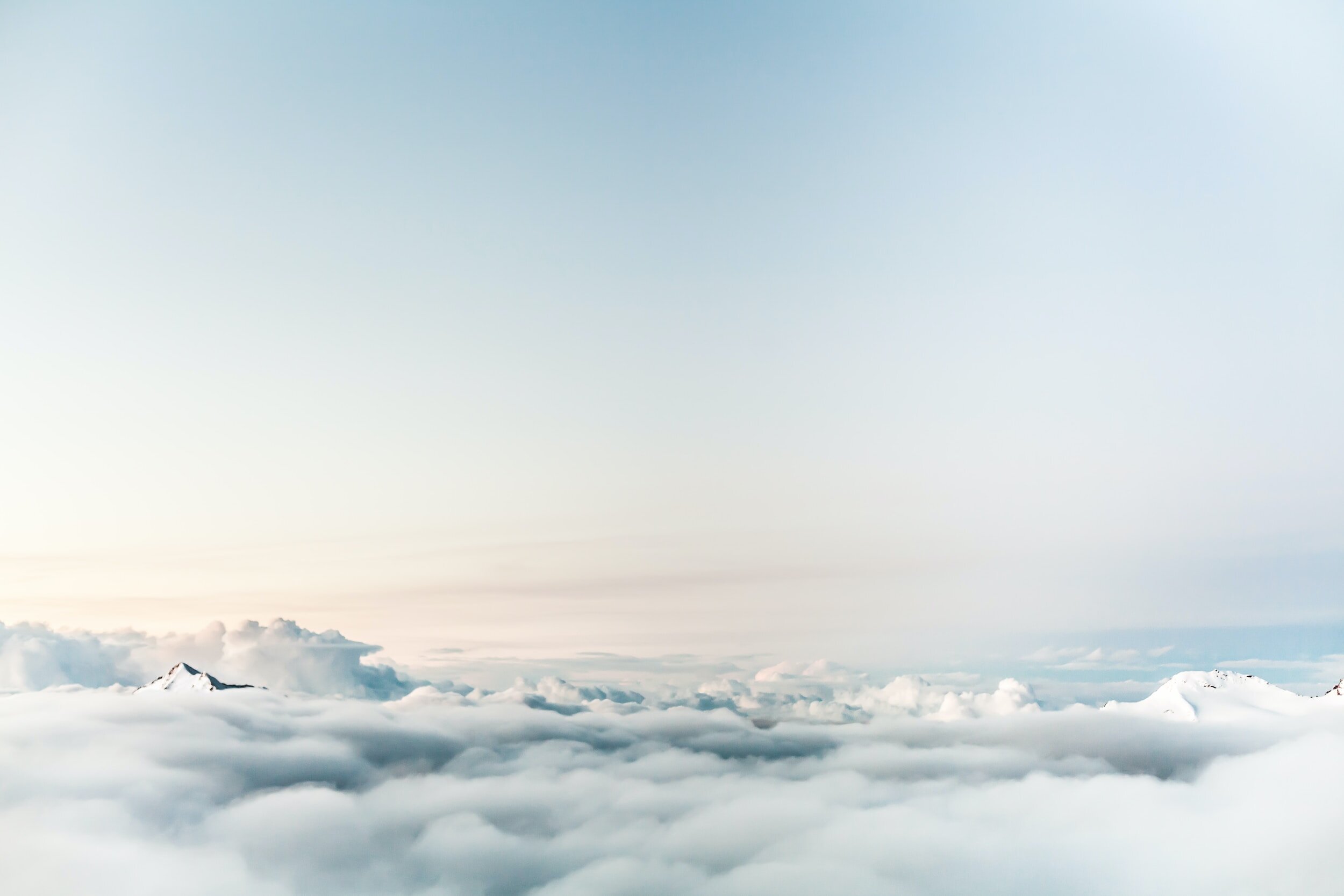
2023 Q2 | Edition 2 | Article 1
The scientist and the engineer
When it comes to solving difficult and intractable problems, two professions stand out with a record of achievement that we can be in awe of: The scientist and the engineer. They may not have the answers to our personal dilemmas, but they can get us to the moon and cure the illnesses that would otherwise have killed us off long before the age we are now.
We don’t need to learn science or engineering to learn from these two professions. Their approaches to problem solving are surprisingly different, and many of the earliest ethical outrages of artificial intelligence come from engineers straying into the territory of scientific enquiry without the scientist’s rulebook to hand.
Many people think that the problem with modern day artificial intelligence is ethics vs machine. It is true that machines can have some pretty unpleasant and unethical impacts. But pure ethics experts can struggle to discuss issues, like where machine bias comes from, because very often the problem isn’t one of human ethics. It starts in a more fundamental place.
When they decided to build machines that could reach some decisions autonomously, they forgot to bring scientific and engineering insight to the problem.
We can learn from those mistakes. Before leaping into a solution of how we think that the world should be built, activating the engineer inside all of us, we might step back first and observe whether we have all the right data and the right handle on the underlying structure causing the problem before we leap in with a solution.
Subjective Objectivity
The ‘truth’ is only ever what we agree on at any given moment. By allowing ourselves to remain open to the possibility that we are wrong, we may get closer to the evidence that will help us to move forwards.
The Scientist
The scientist observes the world, unpacking how it works. Over the years, scientists have realised very wisely that observing the world is an enormously challenging task. Unpacking the underlying structures is even harder. Scientists have evolved a set of principles around how we select little samples of the world to look at. The scientist must take care to ensure that the results aren’t simply found in our little test sample, but are in fact, common to everyone. This isn’t an easy task given that the whole population of the world, or every altitude and landscape of the planet can be challenging to access and assess.
The Engineer
The engineer wastes little time agonising about such issues. The engineer’s role is simple – build models of how things should work. They still go through periods of testing and trialling, but the aim of those trials is not greater understanding and discovery. They’re measure of success is rather more basic – did it work? Did it produce the result I expected it to produce?
AI - where the two problems clash
Now enter the world of artificial intelligence around 2010, when engineers were dominating innovation in machine intelligence. An engineer’s measure of success for face recognition might be that the machine can be shown a set of voice recordings to learn from, keeping a small little subset aside to use to test the algorithm with later. If the AI can perform well in the test later and recognise the words of new voices, it’s a success. Engineers cared little that once they put the voice recognition algorithm in, say, satellite navigation systems, it struggled with female voices.